Winterthur, December 2023 - The medical devices industry looked quite different from today when graduate engineer Daniel Kormann first started working there in 2009. While quality assurance had been established for safe and reliable products, manufacturers were still hesitant to try methods such as cavity pressure measurement. Over the last ten years, measuring this parameter throughout production has become industry standard because it provides reliable information about the quality of both process and product. Equally, digitalization and automation of process monitoring have come a long way. Daniel Kormann, head of business development for Plastics at Kistler, discusses the state of process monitoring and process control in the light of current developments such as AI and new regulations.
Harnessing process data in medical injection molding to enhance quality assurance and efficiency
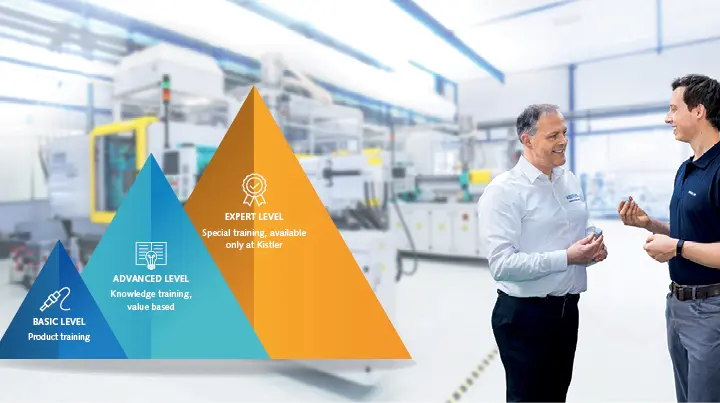
Daniel, what major changes you have witnessed in your career in the medtech industry?
During my time in the medical devices industry, I have seen several major changes. In 2010, when I started out, companies were still skeptical towards cavity pressure measurement. Those that went on to integrate the technology were mainly interested in using it for straightforward process monitoring, ignoring more sophisticated opportunities. When I joined Kistler, one major project was the creation of ComoNeo, a system which was a serious level-up to the previous solution ComoInjection. As a software designed specifically for injection molders, ComoNeo provided a much better user experience and was quickly very successful on the market. It also attracted a lot of interest from the medical field. To meet the needs of medtech companies, we created a company-internal group to unite experts from the areas of plastics applications and advanced manufacturing. While the former bring a lot of material expertise, the latter have extensive knowledge in the monitoring of joining and assembly processes. Joining forces, the team saw the immense potential of gathering and analyzing meaningful data in the medical field. This has lead to Kistler to becoming the sole provider of the process navigator Stasa QC by Stasa, which enables the process monitoring system to provide efficient, automated documentation of the test plans and also to perform the corresponding process analyses. Most recently, we have also presented AkvisIO IME (Injection Molding Edition), our inhouse process data solution to the broader public at Fakuma.
You mentioned quality monitoring and assurance. What are the peculiarities in the medtech industry here?
Quality management and quality assurance is essential in the medical industry as companies manufacture highly sensible products. Regulations such as Good Manufacturing Practice (GMP) in the US and Medical Device Regulations (MDR) in Europe require companies to comply with strict and ever more extensive standards. They have to keep an impeccable paper trail throughout the entire production processes, just as the saying goes: “If it hasn’t been documented, it doesn't exist.” This need for full documentation puts a lot of pressure on companies. It increases the demand for integrated process solutions that both document and optimize all relevant process parameters. The ComoNeo process monitoring system by Kistler, for instance, accurately measures the pressure in all cavities and compares the resulting curve with the nominal curve. ComoNeoPREDICT uses artificial intelligence to predict product quality based on cavity pressure and temperature curves. All things considered, I believe that there is still a lot of untapped potential for digital solutions.
Where are medtech companies already using of digital solutions?
Currently, there are software modules and algorithms that allow injection molders to automatically adjust process parameters if the measurement curve deviates from a previously defined ideal curve. For instance, if the cavity pressure measured by direct, indirect or non-contact sensors is too high or too low, the system can adjust the switch-over point automatically. The Multiflow software module by Kistler balances filling multiple cavities very efficiently. It analyzes fill-time differences between the cavities based on cavity pressure. It then adjusts the tip temperature of the hot runner automatically to achieve a more simultaneous filling. Using automation is becoming ever more relevant as we look at a growing shortage of skilled workers on the shop floor.
Where do you still see untapped potential?
While algorithm-based systems are already in use, there are two areas where we can improve and promote their reception. Firstly, when it comes to convincing companies of a solution they haven’t tried yet it is important to demonstrate that automated systems for the shopfloor are easy to set up and use. We will be working more closely with our customers to learn from their experiences, to improve our products as a whole and, as a result, to enhance their user experience.
Secondly, education is crucial. Educational opportunities are not only helping companies to see the benefits of a digital solution for their specific use case, but also to support employees on different levels in using them. That is why we have established the Kistler Plastics Academy. Here, we support our customers’ digital journey on three levels. On the basic level, we provide training for machine operators, application engineers and mold makers on how to install sensors, how to use the ComoNeo process monitoring system and the data base. On the advanced level, process engineers and production experts learn what is really happening inside the injection mold. On the expert level, data managers and quality engineers get insights into the potential of data management and data analysis.
Artificial Intelligence is a real buzzword at the moment – but how can it help injection molders in their daily production?
One way to make use of AI certainly lies in the analysis of data and automated predictions based on them. The key here would be a state-of-the-art data platform such as Kistler’s akvisIO. It integrates production and measurement equipment such as sensors or field devices and collects their raw data. It then processes it and overall makes data from different sources comparable to each other. Machine learning algorithms can then use the data to draw larger conclusions on the performance of the overall production set-up, to predict maintenance needs and even anomalies. Our vision is that eventually, the algorithm will be able to foresee events that have never occurred before. To make that vision a reality and to also get users on board with the new way of utilizing data, our large team of data scientists focus on usability and user experience in our continuous development. AkvisIO processes and analyzes process data to be directly used as a basis for decision making. This eliminates the additional step of interpretation and makes the data more accessible. Of course, the user can also add their own interpretation of the data to the results based on their own experience. As the need for AI continues to develop within the industry, we’ve set up akvisIO in a modular way. Working with customers and identifying their specific needs will allow us to add features, or specific modules tailored to a particular application or user group. One area of application could be advanced process control including trends and prediction, which could make processes even more efficient and robust, especially in terms of energy and raw material consumption.
Asides from digitalization: Are there any other key topics that impact measuring technology for medical devices at the moment?
Within our focus industries, the circular economy and especially the use of recyclates are currently the most pressing topics. While we don’t see the latter reflected in the medical field yet due to regulations, this could change soon if we can provide a solution which reliably and automatically compensates process fluctuations. When companies start to use larger percentages of recyclates in their manufacturing processes, measuring technology becomes even more important, while it will not change as such. Melts containing recyclates have a fluctuating viscosity and as a result, parameters which indicate viscosity changes such as cavity pressure need to be constantly monitored to ensure a high product consistency. Again, AI could also come into play to detect and even predict anomalies.